Artificial intelligence improves complex operational management processes at ICL’s sites.
The science fiction genre is full of computers and machines that imitate the way people think and behave, and for decades, researchers and scientists have been trying to make such computers and machines actually learn things “on their own.” In the last few years, it appears as though these efforts are paying off and such fictional scenarios are becoming a reality.
One of the most fascinating developments in this field of artificial intelligence (AI) is machine learning (ML), which is a modeling method that enables us to analyze and learn the behavior behind processes and machines based on historical data, to integrate this with real-time data, and to improve our forecasting abilities. The implementation of these complex statistical models requires a large amount of computing power, and for this reason, this approach is based on a combination of smart statistical models and computer science capabilities.
unit at ICL works to identify advanced and innovative technologies that can help the company to improve performance. In this framework, the unit operates in four main areas: the Internet of Things (IoT), wearable devices, autonomous operations, and machine learning
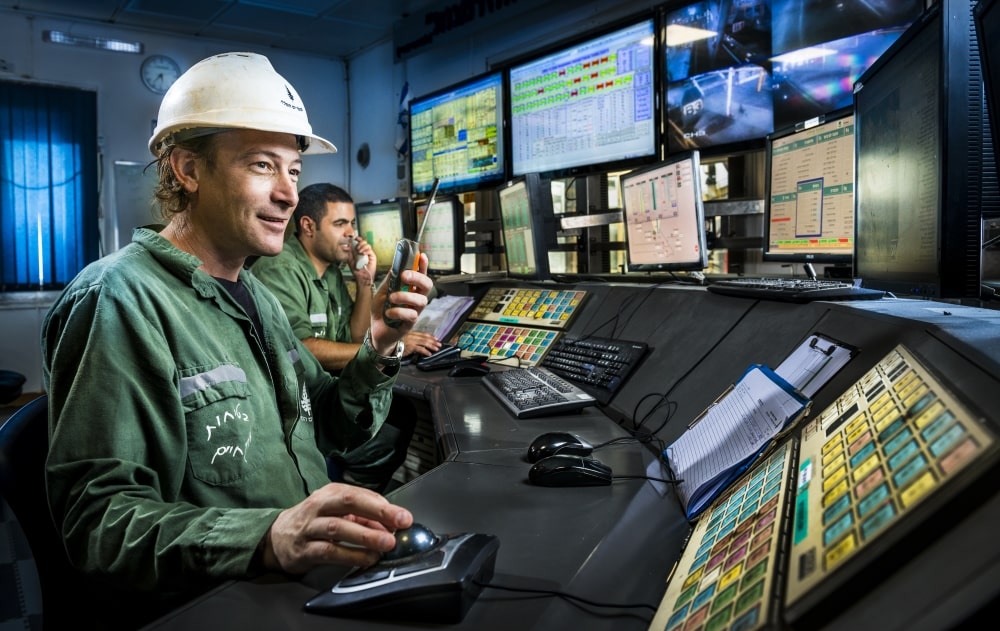
How does it work?
The amount of data in the world in general, and at ICL specifically, is growing at a significant rate. Conversely, only 3% of this data actually becomes valuable information. This presents an opportunity to improve operational performance and move to a predictive maintenance model — one that is intelligently and selectively based on data — while still taking advantage of the huge amounts of accumulated data. One way to do this is by using the machine learning model to predict failures in advanced (Predictive maintenance).
How can this be done? In cases where multiple sensors on machines are used to provide an indication of their working status every minute, they can also be used to proactively monitor the “health” of these machines. If the data are already used to identify the normal working state of a machine, then when it recognizes an irregular data sequence (Anomaly detection), it can predict and give warning about a malfunction. Machine learning enables us to understand what the normal and abnormal states of a machine are at an earlier stage so that the sensors can give real-time alerts about whether the machine is working optimally or not. These alerts can then be translated into a predictive maintenance program to improve machine availability. In cases where the model receives additional feedback from a maintenance team that the machine is actually working fine, the model can learn from its mistakes and in the future, it will not give a warning the next time the situation arises.
You may be interested in:
Industry 4.0: At the Front of the Fourth Industrial Revolution
Machine learning can also be used to improve operational performance. While a traditional operating model is defined with rigid rules, machine learning does not stick to specific rules but rather examines the quality of the decision rules. If the quality is not accurate, it changes the rules to improve the results. The machine learns from the most relevant data and creates updated operating rules that will perform better the next time.
From theory to practice
The staff working in ICL operation rooms needs to make dozens of decisions every hour to control and optimize processes, and their work is based on their knowledge and experience. When it is necessary to intervene and adjust the process, it is reasonable to assume that they have already faced a similar situation in the past, and that it has been recorded and saved in the company’s databases, together with hundreds and thousands of similar scenarios. By using machine learning models, operators can receive recommendations for the best course of action based on the identification of identical situations, actions, and results from past data. In other words, the operational history can be examined in order to recommend that in a current situation (which has already happened hundreds of times in the past), the correct action would be, for example, to raise the temperature.
ICL currently works with companies that specialize in machine learning in the maintenance and operation field and is examining various applications that can potentially leverage its data assets and maximize the added value that artificial intelligence provides. In this context, a number of projects and pilots are currently underway to identify anomalies and predict failures in operational bottlenecks at ICL Rotem and ICL Dead Sea and IP sites.
In the area of operations, projects are being implemented to improve the production yield at the ICL Rotem acid facilities, based on large amounts of operational data. In addition, the company is developing another machine learning model to manage the flow of solutions at the Dead Sea factories. This model combines meteorological, process, physical, and chemical data already available in the databases and together with weather forecasts, processes them in real time and provides a recommendation on how to manage the flow of solutions. The development of the model has been completed and the pilot phase is currently being implemented in the production environment.